Finding focus in the generative AI whirlwind
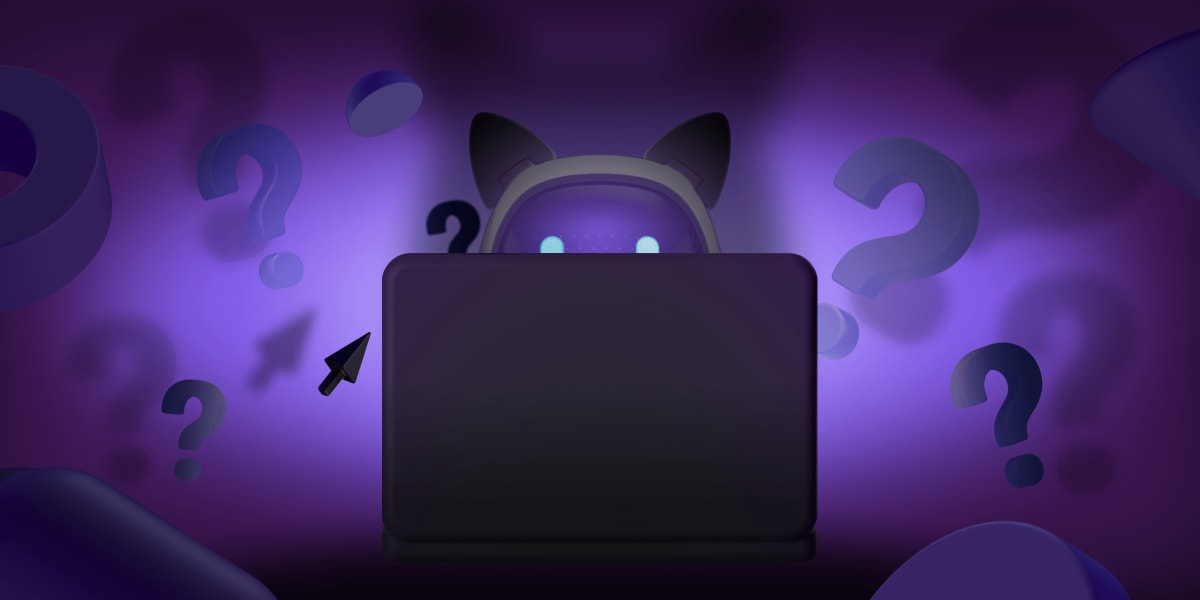
If you work in Product, or in any kind of product-led business, you’ve probably been preoccupied by one thing for the past six months: AI. Recently, it’s transcended the work sphere too — no longer just a topic in board and team meetings and on Twitter, it’s showing up at family dinners, on the front page of major newspapers, and in the halls of government.
In my own work as a product design lead at Pitch, this AI whirlwind has felt both incredibly exciting and overwhelmingly daunting. To help anyone else out there trying to see the forest for the trees, we’re sharing our AI explorations deck. It includes the process and frameworks we used to investigate the opportunities this technology presents, as well as the lessons learned and principles developed along the way.
We hope this will help other product teams, especially those in the generative AI space, deliver value faster. In the spirit of building in public, I’d be really excited to hear your feedback. You can reach me on Twitter at @multikev, or join the discussion in Pitch’s user community.
Fighting the pressure to “ship anything with AI”
The world is in a rush to develop AI features and products right now. Even large incumbents have a fire under them and are innovating like startups. Actual startups are fighting to be associated with the AI gold rush, desperate to avoid being left behind. But at the same time, we’re beholden to the rapidly changing functionality of a handful of central models — from OpenAI, Meta, and Midjourney to emerging open-source models like Stable Diffusion.
As a product leader, you face two opposing risks:
- Rush into shipping features that generate generic, uninspired output, and you’ll probably become irrelevant once every competitor implements the same feature(s).
- Deprioritize AI-powered features to focus on the rest of your roadmap, and it’s likely you’ll be left behind in the technological revolution.
So how do you strike the right balance? How can you stay sane surrounded by the noise about AI? Here are the three lessons we’ve learned, which we’re using as North Star principles as we explore this brave new world.
1 . Generating vs. editing: The balance between human and machine
After spending five years building a presentation tool, we’ve learned a lot about what makes presentations hard. The challenge is not the initial outline or the basic information included, but designing and delivering engaging slides that speak to an audience. That involves collaborating with your team and magically combining things like text, images, layouts, and voiceover to create a smooth delivery experience. It’s a lot more complex than even Pitch’s founders initially thought. Similarly, whatever space you’re working in, it’s a safe bet you have more institutional knowledge about the problems your product addresses than anyone besides your direct competitors.
In our explorations, what we saw very quickly is that AI can help you with parts of the intricate presentation-building process, but that it’s not (yet) sophisticated enough to take over presentation-generation entirely. We’re confident that AI plus a human editor will remain more powerful than AI alone — at least in the shorter term — because of the complexity of the presentation medium.
“AI can help with parts of the intricate presentation-building process, but it’s not sophisticated enough to take over entirely.”
That’s not to say there aren’t areas of our space where AI can be very effective. In fact, even before the current AI boom, there were smaller automated features within Pitch, like AI-powered image cropping.
So ask yourself this: What do you know about the biggest challenges in your space? What elements are straightforward? How is combining them hard? Where can AI enable, and where is human intervention still needed — and likely to be needed for a while? Agree on the answers to these questions as a product team, have confidence in your point of view, and use these answers as the starting point for your strategy.
2. Keeping up with the Joneses: The importance of timing
The question every product manager is asking themselves right now is obvious: How do we ship the right AI-powered features fast? Your marketing team’s probably asking you this as they try to jump on the AI-related news cycle, and your customers are likely starting to wonder too. But there are diminishing returns in pumping short-term leads or signups with AI hype — you could lose those users a month later with a subpar product.
And your challenge goes deeper than the usual pressure to ship standout features fast. The large language models (LLMs) you rely on to deliver these features are rapidly evolving, so you’ll need to factor in external dependencies, over which you probably have no control. Many AI features, like text-rewriting, are becoming as commonplace as bold or italic font options. Image generation will soon become so standard that it’ll no longer be any different than searching the web for images. These types of features should be considered table stakes, not differentiators.
“Instead of asking, ‘How fast can we ship?’ your core question should be, ‘How can we add AI in a way that’s differentiated, offers high value, and speaks to our specific audience?’”
Instead of asking, “How fast can we ship?” your core question should be, “How can we add AI in a way that’s differentiated, offers high value, and speaks to our specific audience?” Otherwise you’re chasing too many opportunity areas, and trying to keep up with too many developments beyond your control.
The lesson here is simple: Avoid copying features others are building without careful reflection, and don’t prioritize speed over strategy. Instead, filter all your development options for AI products and features based on 1) Uniqueness and defensibility, 2) Value to your target audience, and 3) Current and near-future expectations of your target audience. If you rank all your options based on a mix of these factors, it will guide prioritization and help you focus.
3. It’s a marathon, not a sprint: AI success depends on fundamentals
Some companies are better positioned to ride the AI wave. Advantages include having a modern web stack, access to large amounts of data that assist LLMs, and a nimble, highly adaptable team. It’s time to ask yourself what your organizational advantages are when it comes to AI. That said, adding value in the age of AI still involves good product fundamentals. Teams need to stick to design sprints, follow clear roadmaps, and rigorously prioritize which problems to solve.
There are also AI-specific considerations. To build long-term, differentiated value in your space through AI, you’ll need access to data to train your own AI models. What data do you have that others don’t? Where is the data? Is it usable? Answering these questions is key.
Team structure can help balance some of the opportunities and distractions that AI offers. At Pitch, we’ve created a dedicated “moonshot” team who can focus on incubating solutions to the biggest, thorniest presentation problems using AI — without distracting the teams delivering the majority of our roadmap.
Ultimately, you need to think hard about whether you’re putting measures in place to succeed in this new context. Product fundamentals didn’t go away the day ChatGPT came to town. But you may need to restructure or rethink processes, data structures, and team organization to balance risks, rewards, and focus.
Where these lessons have led us
One thing we’ve always been sure of is that the world doesn’t need more mediocre presentations. There’s a real danger in focusing on the wrong AI opportunities, because it could result in exactly that.
“The world doesn’t need more mediocre presentations.”
Our product team works in six-week cycles, so we ran a hackathon within one cycle to understand our AI opportunities, research the tech, and prioritize work based on all the criteria outlined in the lessons above. We then had a single product team explore these in more depth — you can discover the output of our explorations in this deck.
Now, we’re pursuing two parallel AI paths:
- Commoditized AI APIs as a feature development tool. We’ll continue to consider publicly available APIs as tools to enable feature development. We won’t search for problems just to be able to use a specific technology; instead, we’ll apply the technology, if appropriate, when solving the problems our current and future users care about.
- Foundational AI models as a differentiator. Instead of passively awaiting new models alongside competitors, we’ll lead the way in collaborating with infrastructure providers and model makers. We’ll combine our domain knowledge with their technical expertise, and solve the biggest challenges involved in building great decks. This will create new opportunities for differentiated and defensible features.
Have any thoughts about our explorations, or any opinions about what would work best for Pitch users? Update: We’ve since launched Pitch 2.0 with brand-new features, including a free AI generator to help you jump-start your decks. How are you approaching generative AI as either a user or product leader? Tweet me at @multikev, or join our community to continue the conversation.